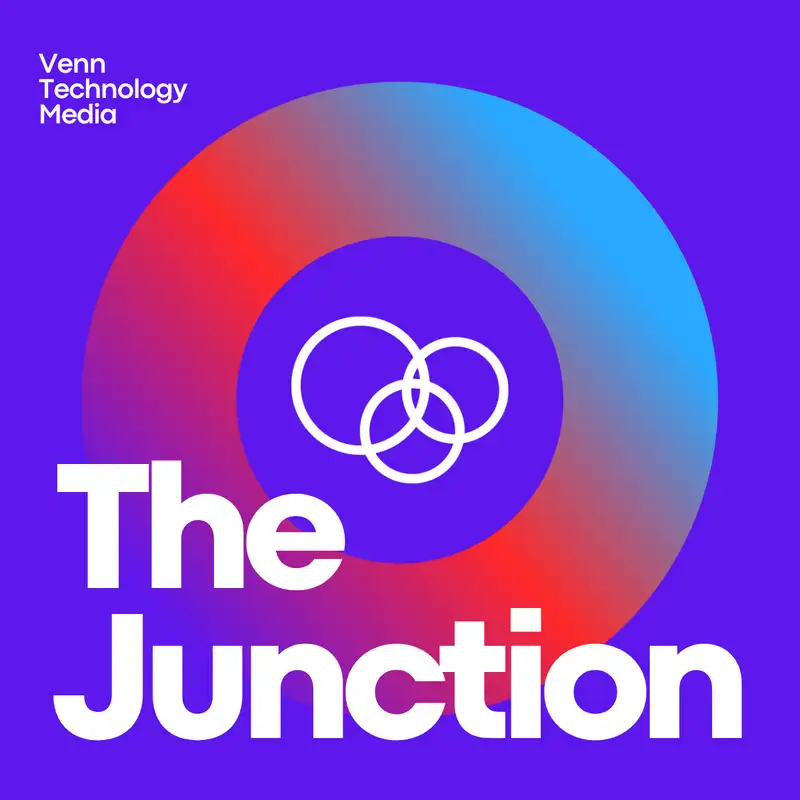
#18: Keeping Humans "Near the Loop" with Jerrold Jackson
Welcome back to another episode of the Junction.
We are excited to have Jerrold Jackson
here with us today.
We got introduced to Jerrold through our founder.
He networked us up because we were talking
about these things called AI and ML.
And then we met Jerrold and realized
we were about ten years behind.
So, Jerrold, tell our listeners who you are, what
you do, what your background is, and then we'll
kind of get into some more of the nitty
gritty what you're doing with AI and ML today. Yeah.
So I'm Jerrold Jackson.
I am, broadly speaking, a
technology and data entrepreneur.
I've got a really mixed background.
A few careers ago, I was a psychotherapist at
an outpatient psychiatry clinic in New York City.
And then fast forward, a PhD business school and
about 15 years of technology experience later, I find
myself now leading a fitness and wellness startup based
in Houston, Texas, but also advising pretty widely across
the health and wellness space.
Slightly.
I'm curious, in the background, right, going from
kind of medicine then to business now to
technology, was there always this underlying theme of,
like, I mean, it sounds like in each
of those you just want to help people.
I don't know if you maybe felt that way,
but is there an underlying theme on that front?
There is, yeah.
The two themes are I want to have an
impact and help people, but I also want to
use data and technology to do that.
So what I failed to mention is even
going back to my undergraduate days, I was
actually it support at my undergraduate college.
So I was a nerd.
I mean, my father and I were building the
big computers with the big boxes and the towers.
I was building those as a kid,
was doing all kinds of things.
I convinced my parents to buy
multiple AOL dial up lines. Remember that?
Like, way, way back. Throwing it way back. Yeah.
So I was in all kinds of really cool stuff back then.
Was just like kind of early,
early stage Internet and web.
Built my first website when I
was ten, that kind of kid.
But then fast forward, even into college, like I
said, I was doing kind of tech support things,
was learning about early, early stage kind of statistics
and advanced statistics, and then eventually machine learning.
Even when I was doing psychotherapy work,
my clinic ran lots of clinical trials.
And so I became interested in not
only implementing the evidence based intervention, but
also running some predictive analytics on why. Right.
Why is something happening and what might happen if.
So, my phd was from Mount Sinai School of Medicine.
Of course, the advanced statistics work I did,
there was a great parlay into more of
the machine learning then, you know, moving into
industry, and even through business school, started to
do some machine learning based work.
Initially, the industry was actually for a hedge fund.
So I started kind of in that world,
which is highly regulated, or at least the
work we were doing was more regulated.
It was more financial services on behalf of the
hedge fund, and then, of course, into the wild,
wild west of health and wellness and worked in
healthcare payments for a little bit.
Had my first startup company in the health
and tech world about 13 years ago with
a colleague of mine at Mount Sinai.
Exited that company a while back.
But yeah, I've always been interested in kind of woven
impact and data through all that I do, man.
Tell me, obviously you've got this diverse background.
I think we're going to talk about some of the
studies that you've done or the work that you're publishing.
But I'm just curious, what's your take on the
last few weeks with all of the, maybe I'll
call it not like government politics, right?
But Sam Altman's out.
Anthropic does a big push right after that happens.
I don't know, what's the current sentiment, at least
from you, like in your mind, for December?
Yeah, it's a great question.
I look at all these big themes, right?
Thematically, blockchain was huge when it first
launched, and it tried to position itself
as, like, how is it practical?
How does someone actually use this technology to
make money, to make something more secure?
All those kind of things.
I put generative AI in a different bucket, but a
similar workflow, which is that people are still trying to
figure out an enterprise, how to use this to make
money or to make someone's life easier.
A lot of folks, there's lots of sentiment
around, am I going to lose my job?
But really it's like, what's the practical application of
this and the Sam Alton kind of movement?
First, he's fired things back and
all that kind of stuff.
That's just, to me, it's details, right?
It's kind of just this new technology
trying to settle itself in a marketplace.
I have to remind people that large language models
may be new, this transformer network that GPTs are
built on may be on the newer side of
things, but language models are not new, right?
Natural language processing is not new.
So as we think about, AI is now
synonymous with chat, GPT, or cloud bianthropic.
These newer large language models.
AI is synonymous with large language model, but
language models are not new at all. Right?
There's a whole field of natural language processing
that's been around for a long time.
What's new is cloud computing resources.
And I've got a sneaky suspicion,
chase that down the road.
We'll probably actually see a bit of a
reversion to the mean, where it's like, oh
my gosh, there's too much access.
It's too available, it's too
scary, it's too understood.
How do I go back to my private cloud? And then.
I'm not saying that we're going to be back
to on prem support, but it's almost like you
see these cycles where people get really excited.
They adopt these early adopters, something big happens.
People say, oh, crap.
Or maybe something a bit more explicit than that, and
then it reverts back to something even more secure.
You talk about people wanting to go back.
I don't know how far back people want to go, but
I have been known to tell you, Chase, that I would
go back to the days of a landline sometimes because I'm
so overwhelmed with all of the availability that we have.
It's a blessing that we've got all
these incredible technologies that make it.
People can work from wherever. Right.
But then I just think about, man,
wouldn't it be nice to just.
I just wasn't at the house.
Sorry I missed your call.
Sorry, I didn't get your text.
I think it would be great.
I think it would be great.
And I think what comes to mind in terms of old
technology that's currently having its heyday is the QR code.
Right.
The pandemic brought the QR code to us.
That's not new. Right.
But they're finding new applications, which
I think is really funny.
But it's a fairly secure way to transmit
information to understand who someone is, et cetera.
So I agree with you.
I think that chase, to your original question, I think
the last month has brought us maybe only the beginning
of what we'll see probably through the next, I'd say,
a handful of quarters at this point.
Some new release people are chasing Agi. Right.
Like some new application of what we're
trying to do will come out.
The one theme I'll say that I think is really interesting
is both in the work I currently do and in some
work I'll be writing about soon and publishing soon.
I think that doing this responsibly
is going to be the key.
So I think partially how this plane
lands at Enterprise and at scale is
doing all this generative AI work responsibly.
Yeah, you bring up a great point.
Mel and I were talking about
predictions for the next year.
And one of those, at least in my mind,
is people are going to be utilizing the technology
in a way where they're not really going to
talk about the underlying structure of what they're doing.
They're just going to come to
market with a new product.
And I think we maybe see a little bit about
that with how the large language models are trained.
Right.
Where are they getting the data set from? Right.
And it seems to be like, well, they just
scraped the entire web, and maybe there's some moral
implications behind that, but they did it.
And here we are.
And going back to your idea of the reversion of
the mean right now that their models and the weights
are out there for you to download, we have some
colleagues internally that are running these models on their servers
at home, and we've got all the vpns set up
right, and we can just play around and we're not
racking up giant electricity bills.
So I can see it going a ton of ways.
Actually, we were just, like, running cost
analysis to figure out relatively inexpensive gpus
that most gamers use a whole lot
less electricity if it's already trained. Right.
You're just kind of utilizing existing power, and maybe
you're ramping up the electricity bill a little bit,
but we're talking like maybe a couple of month.
I'm curious if, as we kind of pivot to
how your team and the folks that you are
working with and the different initiatives that you're working
on, are you bringing, where do you sit on
that spectrum of responsible use, and how are you
incorporating that into the work you're doing currently?
I think about this a couple of different ways.
As a technologist, I like solutions that
are less monolithic and more modular.
Those aren't complete opposites.
But what I mean by that is a monolith,
broadly speaking, is something you build for one purpose.
More of a modular architecture is an architecture are, that
literally is built as a sum of multiple parts.
And you can swap out this part
and optimize it whenever you want to.
You can swap out this part and optimize it.
Oh, this part over here failed. That's okay.
The whole system didn't fail.
You can swap out that one part.
So the way I think about this
to date has been very modular.
And the LLM component of any solution, broadly
speaking, any automated solution has to be modular.
So being LLM agnostic first and foremost to me
has been critical in launching products at scale, particularly
products that are b to b, because different businesses
may have a different perspective on what large language
model they want to use.
They may have a preference,
for example, cloud by anthropic.
By the way, I don't get paid
by any of these folks, right?
But cloud by anthropic is one example of
one that has been pretty forthright about.
They actually published white papers about
how they're doing, what they're doing.
And they've been very transparent and they kind of
have gone to market as this responsible, trustworthy LLm.
Others have not been quite as transparent
about what they're training on, how they're
training, their model, et cetera.
All of these can be considered a foundation model.
So as you all were kind of alluding
to, in my case in particular, kind of.
So far, I've launched these different solutions in an LLM
agnostic way, which means I can swap out llms as
I want to in a very modular sort of architecture.
And then I can very responsibly, based
on the use case, deploy this technology.
So, for example, a marketing copy use
case, people refer to llms as hallucinating.
It may actually be more of a feature versus a bug.
I want extreme creativity for marketing. Right.
Give me the top 5000 examples of
how to take these three concepts.
If I'm prompt engineering, take these three concepts or
this bucket of words and give me 5000 different
versions of sentences or whatever the use case is.
If I'm recommending some very specific actions
for someone to take, however, I don't
want that level of creativity.
You can actually ramp up and ramp down.
They're called hyperparameters.
How creative you want these llms to be?
Another way to make these things responsible is
to pair them with a recommendation engine or
some more structured way that you actually want
to deliver actions and sort know next steps.
So this is one critique I have about
some of the ogs in the recommendation space.
So take, for instance, Netflix.
Netflix is a prolific company with a
prolific recommendation engine along the lines of
Amazon prime or other companies.
These big, big, massive companies
worth billions of dollars.
These recommendation engines, which are not gen
AI, they primarily recommend that you just
consume more, buy more, watch more.
I've never had Netflix say, hey, hold
on, brother, you've watched too much today.
Or, hey, Amazon prime, you've bought too
many peepee pads for your dogs.
They're driving consumption, consumption, consumption.
Whereas I think a more responsible way to leverage, let's say,
gen AI is to, instead of just have it drive a
conversation kind of into a hole and potentially into a direction
that you don't really want is to pair it with a
very intelligent way of recommending something to someone in a way
that is a bit more guided and might even kind of
say, hey, maybe calm down today, maybe don't do so much
today, don't do the most today. Right.
So there are some responsible ways to leverage Gen AI,
and I think it really depends on the use case.
I like that thought a lot.
I even see some of this.
I don't know how much you've played around with Chat
GPT, but they have different versions of the same model.
And from version to version, maybe it gets
better or maybe it gets worse, right?
And I think a lot of in the
background they're trying to manage their electricity bill
just to be real broad, but also provide
more functionality, but at a lesser cost.
So they're constantly adjusting their weights or whatever,
but the end user doesn't know the difference.
Oh, you're so right.
Yesterday we were talking about the trend
around mental health and people using these
models as sort of therapists.
And one of the benefits that's
being touted is like the accessibility.
It's there all the time, you can always talk to it.
But hadn't really considered the possibility of like,
maybe there is such thing as too much
consumption and something that can help kind of.
We were talking about, well, with a therapist or
a counselor, you're kind of locked into your 30
minutes or your hour for that week.
But if it's Chat GPT or Claude, they're there for you.
So I'm sure there's pros and cons
on both sides, but I think that
idea around consumption is really interesting.
Well, you have this high level of like, well,
now you're talking to the therapist GPT or you're
talking to the coach GPT and different personalities almost.
But under that you could be flipping out models based
on how much knowledge we need this personality to have.
And you can make it a feature, right?
Like, well, here's the expert coach, right, that
knows everything about everything, but maybe you're paying
half as much and now you get, I
don't know, the high school coach.
I can see use cases across the board
and I too wouldn't call them bugs, right?
They're features.
It's providing a level of functionality to somebody
while at the same time flipping out.
Like the modular idea that you had where you can
flip out a model but not interrupt that user experience.
Just like you were talking about, Gerald.
Yeah, I think Chase, to your point earlier
too, about GPT 3.5 versus GPT four and
different versions and they'll have it.
I know OpenAI is working on a
version five right now as well.
If we're talking about OpenAI
specifically, what's tough about that?
If you're just purely going through OpenAI to
build an entire product, and once again, I
don't get money, nor do I don't get
anything pros or cons from speaking about it.
But just me observing as a consumer and someone
that builds products using these things, it is kind
of terrifying because literally they could have an announcement
tomorrow that says that they've come out with GPT
five and they're downgrading 3.5.
You don't know what that means.
Is the knowledge base changing?
Is the word vectorization strategy
on the back end changing?
If and when you're working with a product or a
solution that's not as transparent, that's what you get.
On the other hand, there are the major cloud providers,
Microsoft Azure of course, as being the main one.
They're serving up these models, really all the major
ones, cloud, GPT, et cetera, they're setting them up
as managed services, meaning you pay those companies for
a stable version of those models, that is not
going to change as OpenAI kind of wishes.
So in doing so, what you get is if you go
through Azure and pay a little bit more money, you get
a more stable product that you can rely on.
Well, I don't know how close you stayed to the dev
day, the OpenAI dev day I put my application in, but
I'm apparently not, I was waiting on my press pass.
Yeah, we're not well known enough
to get access to that.
But within that one day, right, they blew all of
the chat bots that have popped up out of the
water and just, I mean, maybe didn't completely put them
out of business, but I know I saw several Reddit
posts that were like, I just lost my entire business
that I've been working on for the past six months.
And it's like, yeah, well, yeah, when the technology is
shifting that much, you have to be prepared for that.
But I like your thought on the azure piece, right?
It provides a level of stability for
the SMB space like the enterprise.
Hey, I want to build a product off of this, but
if you want bleeding edge, you got to be prepared to
go off the edge almost, because tomorrow it could change.
GPT five might do everything that
we're already talking about and more. Yeah.
And I gave the blockchain example.
We saw the same thing in crypto. Right?
So if you were invested in bitcoin
or ethereum, you're great big, stable.
If you have one of the meme coins, you might
be able to afford a new shirt on one day,
and you might lose two shirts the next day.
It's all over the place.
It's exciting, but I think it also kind of facilitates
for me, this is what I'm writing about these days.
I'll say as well, in general, and this is
kind of a general place to find these things.
Ww dot neartheloop.com.
Near the loop, as in humanintheloop.
So L-O-O-P.
So, neartheloop.com is where I'll publish
these musings, kind of over time.
But my thought is the following.
Long before chat, GPT, and even OpenAI kind
of changed the game, really, this year.
Last couple of years, there's been a lot of
thought around AI systems without a human in the
loop, and I think there are a number of
industries where that can certainly work.
Once again, I mentioned the idea of marketing copy.
I'm not a marketer, and nothing against marketers,
but there's a certain world where you can
generate a bunch of copy really fast.
Do I want my attorneys to be billing
me a billable hour, but using GPT to
spin up legal documents, probably not, right?
So I want a human in that loop for sure.
Do I want my local doctor's office using
some GPT based or enhanced radiology technology?
No, I probably don't. Right?
I want an actual human.
It's okay if a machine takes things a
certain percentage of the way with certainty, but
I still love a human in the loop.
Well, the concept I have is, what if a human
was near the loop, maybe not in the loop the
whole time, and maybe not fully autonomous, but what if
a human was near the loop to where, especially in
some regulated industries or industries where the stakes are pretty
high, you have a built in mechanism to where you
have a human who can still make sure know the
actual machine or the bot is still on the rails.
Because I think it's funny, last time I was
in San Francisco, I tried the autonomous driving cars.
I applied.
I was on a list for Waymo for a couple years, got
off the list recently, tried a waymo for the first time.
It was awesome.
But anyone that's been to San Francisco knows that you oftentimes
see a human in the driver's seat of a waymo.
You're like, wait a minute, I
thought it was self driving.
There's still occasionally a human, and
there's articles published about this both
academically and non academically.
There are still occasionally humans
in that loop, right?
There are humans in the driver's seat who are
assisting that vehicle's data collection with all of its
thousands of cameras, because we still can't quite get
away from a human giving feedback.
Yeah, I always do a gut check with chase.
Every so often, I'll play this game with him.
Like, let's go back and forth and list the different
things that can't be automated or taken over by AI.
And I still have, like, there's those,
like, fly fishing or being some kind
of in person yoga instructor or something.
Like, the things, to your point,
Gerald, that you were talking about.
Like, we still desire to have a human near the
loop, even if we know additional insights or data.
Because I think even when I brought up
the fly fishing example, you're like, well, what
if I had the ability to.
It could correct my movements and stuff. Great.
But you're the one still doing it.
You're still a human near the loop or in.
I don't know, maybe I'm not using the right.
No, that's example for that.
But it's just a different use case.
I don't foresee anybody absolutely loving the day
where they don't have to interact with anybody
whatsoever, but there's also nobody to interact with.
Man on the moon, all by themselves, totally disconnected,
and all they've got is their large language models.
I don't somebody being like, give that to me any day.
I'll do it right now.
On average.
What about when the robot is talking to another robot?
The agent idea, the auto GPT, some of
these GitHub repos, right, that are trying to
get the large language models to talk to
each other before they ask for a recommendation.
Do you see that really exploding and
really being, like, a really big deal?
And now you've got, like, 20 different
agents, right, working on a project?
Or is it really just in your mind going to be
like, yeah, there's one agent, one super strong agent, and does
most of the work, and you have the human next to
that agent, and they work side by side.
I definitely see a world where industry, right?
We're going to try it.
We're going to try this agent to
agent set of interactions at scale.
I know it's being tested, and I'm certain that there
are industries where this will make a lot of sense.
I see that entire sort of collection of
efforts hitting a ceiling at some point where
there's some pretty measurable negative impact.
And I mean, not just people losing their jobs.
But once again, long before generative
AI, there have been concepts of
reinforcement learning, for example, right?
It's a basic behavioral kind of concept rooted in
all sorts of behavioral theories where with the right
reinforcements in place, effectively, this is not an official
definition for my behaviorist out there, so don't pin
me to the stake on this one.
But if you have a series of consequences built
in, both negative and positive, you can teach a
machine, or in this case a bot or an
automated system to almost learn over time.
There's some famous examples of people attempting this
work and then kind of on the side,
hush hush, secretly teaching it rules to expedite
the process of learning through reinforcement.
Because true reinforcement learning can take, like in a
fully automated way, can take a really long time.
It's not very efficient.
But I do think that there will be
industries where we will attempt to have agent
to agent and maybe even multiple agents interacting.
But I think it will hit a ceiling at some
point and people will realize that for interactions that have
actual consequence, like real day to day consequence, we will
need to have a human near the loop.
That makes a lot of sense too, right?
Fully automated, too much risk, right.
It could just explode and your whole business
model goes under, out of the loop.
Or maybe like, no, AI is maybe not necessarily
the best either because now your competitors are going
to find a way to use it.
But in the loop where the AI is doing enough
work to expedite, what you're doing actually scales, right?
Because now you can have these agents or
whatever they end up being, help you do
your work or elevate these things and makes
you faster or better than your competition.
And in the business world, not that customers aren't
our primary focus, but if you're not in business
because your competitor puts you out of business, you
really can't help a whole lot of people, right?
So it's in most businesses interests, right, to pick up
this stuff, start to learn it, start to figure it
out, at least be aware of what's going on.
But Gerald, I've got to ask.
I know most of us, definitely, you
deal in the objective world, like statistical.
Give me the numbers.
Let me look at the data.
But you mentioned something that
I think, well, the rumor.
So here we're going subjective for a second, right?
But the rumor was that Sam was out because
they developed a model that can learn, right?
And maybe the rumor was it's not super
crazy, but it demonstrated the ability to learn
without rules or sidebars or things like that.
So I'm curious, are the rumors just totally not
a thing because it's not even possible yet?
Or is there a chance that they came out
with something, or they've built something that can learn?
And maybe it's rudimentary, but if it can
learn at all, and it can learn at
any rate whatsoever, that's game changing.
What are your thoughts?
Yeah, so once again, I think that
the core of this is reinforcement learning.
There's even reinforcement learning
with human feedback.
These are all things that for a while now, current
and former employees of really kind of big tech, right?
So meta and Alphabet have published
on these for a long time.
There's really a few players, the two of them
and maybe a couple of others, that actually have
the data sets big enough to train these massive,
massive foundation models on billions of parameters.
The human feedback part typically comes into play
when you have some expert level feedback, right?
But the use cases get really nuanced.
You can't collect human feedback on
every topic known to man, right?
That's just something that's not even feasible.
So I definitely think the technology is
there to give this general purpose foundation
model that can learn autonomously.
I definitely think the technology is there.
The data sets are there.
My concern still is in the nuances
and specifically the nuanced use cases.
So once again, do I want
an autonomous system doing my taxes? No.
Do I want autonomous system even making my coffee?
Heck no.
I think there are still some specific use cases
where, depending on, at the end of the day,
things that we as humans really, really care about.
And there's a long list of
them, and they're all individualized.
Those are the things where I think that, as
a professor of mine said many years ago, models
are beautiful, but they're not real people.
So we're still in that realm where we've got
models that can do really amazing things, but at
the end of the day, they aren't real people.
That sounds a lot of like what we were talking
about the other day, is that original content is king
from a market, like in the marketing space.
I don't know if you'll ever be
able to really, truly replace the humanity
in humans with something like Agi, right?
Because maybe they won't ever get there.
I won't say never, but I think there's
a long stretch of time you can get
pretty far now with deep fakes, right?
I've seen some pretty convincing deep fakes.
And if you use that as a medium to deliver
a message in a very visual way, combined with, let's
say, a script that's either generated dynamically or previously.
You can do a lot to communicate that.
But I think we also run up against regulation, right?
So our current administration has put out probably the
biggest, most opinionated piece on how some of this
might begin to shape up in terms of regulation,
and who knows where that's going to go.
I'm not going to touch that topic with
a ten foot pole, but I think that
regulation will likely come into play here.
And there seem to be a few lines in
that sort of initial regulation regulatory piece that could
potentially put some pressure on deep fakes, for the
primary reason, of course, preventing bad actors from convincing
people that something is real when it's actually just
generated by some automated system.
You're reminding me of something that I was thinking about the
other day, how I think of the Cold War, right?
And it was a race to arms, right, the nuclear arsenal.
And not to be drastic, but we're definitely on
a race with other countries to get this technology
harnessed to figure out how to use it well.
And whoever is really first to beat the
others may never get out of first place.
So there's a whole geopolitical thing going on there that we
probably don't have a ton of time to talk about.
But I'm curious.
You obviously know what you're talking about.
You've got a ton of experience in this space.
If I'm a listener wanting to find more out about
you and what you've done, do I go to LinkedIn?
Are we on TikTok? Where should I go?
Find more about what you've done in your
research and at work and things like that.
Yeah, I'm not nearly cool enough to be
on TikTok, so I'll just start that.
Definitely easy to find on LinkedIn
or just googling my name.
So Gerald M.
Jackson or Gerald Jackson.
J-E-R-R-O-L-D. Jackson?
Geraldjackson.com leads to my LinkedIn, so that certainly
is handy, as I said, as well.
Certainly heading into Q one of 2024 and
ongoing, I'll be publishing a lot of my
thoughts here on the near the loop concept. So.
Www.neartheloop.com.
I think there are pretty easy applications in
the world that I currently play in the
sort of health, wellness and fitness world.
And I think certainly beyond, as you think about things
that people care about, financial services, how we travel from
point a to point b safely, et cetera.
Well, we look forward to continuing to
follow what you do on this topic.
Gerald, I know this has been
a really fun conversation for us.
We geeked out on our intro conversation prior to that.
So thank you for spending the time with us.
And we look forward to, like I
said, seeing where all this stuff goes.
We'll probably have to check in with
you at some point next year. Totally.
We'll have you back.
We'll have you back and we'll figure out how much
of our predictions were right and how much they were
so far off because we weren't thinking that far.
Gerald, thanks for joining us.
Everything that we've talked about today, like Mel said, is
top of mind for a lot of what we've got
going on in house that we're doing for our clients.
If you're listening today's episode, check out Gerald on
LinkedIn and give us your thoughts and feedback.
We'd love to hear what you guys have to think,
but until we see you again, keep it automated.